Обсуждение: Questions about btree_gin vs btree_gist for low cardinality columns
I have been hoping for clearer direction from the community about specifically btree_gin indexes for low cardinality columns (as well as low cardinality multi-column indexes). In general there is very little discussion about this both online and in the docs. Rather, the emphasis for GIN indexes discussed is always on full text search of JSON indexing, not btree_gin indexes.However, I have never been happy with the options open to me for indexing low cardinality columns and was hoping this could be a big win. Often I use partial indexes as a solution, but I really want to know how many use cases btree_gin could solve better than either a normal btree or a partial index.Here are my main questions:1."The docs say regarding *index only scans*: The index type must support index-only scans. B-tree indexes always do. GiST and SP-GiST indexes support index-only scans for some operator classes but not others. Other index types have no support. The underlying requirement is that the index must physically store, or else be able to reconstruct, the original data value for each index entry. As a counterexample, GIN indexes cannot support index-only scans because each index entry typically holds only part of the original data value."This is confusing to say "B-tree indexes always do" and "GIN indexes cannot support index-only scans", when we have a btree_gin index type. Explanation please ???Is it true that for a btree_gin index on a regular column, "each index entry typically holds only part of the original data value"? Do these still not support index only scans? Could they? I can't see why they shouldn't be able to for a single indexed non-expression field?2.Lack of index only scans is definitely a downside. However, I see basically identical performance, but way less memory and space usage, for gin indexes. In terms of read-only performance, if index only scans are not a factor, why not always recommend btree_gin indexes instead of regular btree for low cardinality fields, which will yield similar performance but use far, far less space and resources?3.This relates to 2. I understand the write overhead can be much greater for GIN indexes, which is why the fastupdate feature exists. But again, in those discussions in the docs, it appears to me they are emphasizing that penalty more for full text or json GIN indexes. Does the same overhead apply to a btree_gin index on a regular column with no expressions?Those are my questions.FYI, I can see an earlier thread about this topic (https://www.postgresql.org/message-id/flat/E260AEE7-95B3-4142-9A4B-A4416F1701F0%40aol.com#5def5ce1864298a3c0ba2d4881a660c2), but a few questions were left unanswered and unclear there.I first started seriously considering using btree_gin indexes for low cardinality columns, for example some text field with 30 unique values across 100 million rows, after reading a summary of index types from Bruce's article: https://momjian.us/main/writings/pgsql/indexing.pdfThis article was also helpful but yet again I wonder it's broader viability: http://hlinnaka.iki.fi/2014/03/28/gin-as-a-substitute-for-bitmap-indexes/Thank you!Jeremy
Jeremy
On May 29, 2019, at 10:59 AM, Jeremy Finzel <finzelj@gmail.com> wrote:On Fri, May 24, 2019 at 10:25 AM Jeremy Finzel <finzelj@gmail.com> wrote:I have been hoping for clearer direction from the community about specifically btree_gin indexes for low cardinality columns (as well as low cardinality multi-column indexes). In general there is very little discussion about this both online and in the docs. Rather, the emphasis for GIN indexes discussed is always on full text search of JSON indexing, not btree_gin indexes.However, I have never been happy with the options open to me for indexing low cardinality columns and was hoping this could be a big win. Often I use partial indexes as a solution, but I really want to know how many use cases btree_gin could solve better than either a normal btree or a partial index.Here are my main questions:1."The docs say regarding *index only scans*: The index type must support index-only scans. B-tree indexes always do. GiST and SP-GiST indexes support index-only scans for some operator classes but not others. Other index types have no support. The underlying requirement is that the index must physically store, or else be able to reconstruct, the original data value for each index entry. As a counterexample, GIN indexes cannot support index-only scans because each index entry typically holds only part of the original data value."This is confusing to say "B-tree indexes always do" and "GIN indexes cannot support index-only scans", when we have a btree_gin index type. Explanation please ???Is it true that for a btree_gin index on a regular column, "each index entry typically holds only part of the original data value"? Do these still not support index only scans? Could they? I can't see why they shouldn't be able to for a single indexed non-expression field?
2.Lack of index only scans is definitely a downside. However, I see basically identical performance, but way less memory and space usage, for gin indexes. In terms of read-only performance, if index only scans are not a factor, why not always recommend btree_gin indexes instead of regular btree for low cardinality fields, which will yield similar performance but use far, far less space and resources?
On 2019-05-30 10:11:36 -0700, Will Hartung wrote: > Well part of the problem is that “B-tree” is a pretty generic term. Pretty much > every index is either some form of hash, or some form of tree. We use the term > B-tree, even though pretty much no major index is actually a binary tree. There seems to be no consensus on what the "B" stands for ("balanced"? "Bayer"?), but it definitely isn't "binary". A B-tree is not a binary tree. > They almost always have multiple branches per node. Yup. A B-tree is optimized for disk I/O and uses large (one or more disk blocks) nodes with many children (and often a variable number to keep the size of the block constant). > It’s also important to note that over time, while we may use the term B-Tree > generically, there are many specialized trees used today. There are several variants of B-trees (B+, B*, ...), but B-tree is not a generic term for any tree. A B-tree has specific characteristics. https://en.wikipedia.org/wiki/B-tree#Definition follows Knuth's definition. > Historically, the conventional B+Tree is popular for database indexes. A B+Tree > rather than relying on “nodes” per se, really is based on pages. Nodes alone > tend to be too fine grained, so it’s a game of mapping the nodes to pages on > disk. In the case of a B-tree a node *is* a page (or block or whatever you want to call it). A B-tree uses large nodes with many children, it doesn't cluster small nodes together. [...] > In a GIN index, if you index “ABCDEF”, there will be a A node, B node, C node, > D node, etc. (And, please, appreciate I am talking in broad brush generalities > here for the sake of exposition, not specific details of implementation or > algorithms). So, simply, by the time you get to the bottom of an index, all you > find is the pointer to the data, not the actual key that got you there. The Key > is the path through the tree. You probably have generalized too much to answer Jeremy's question. Firstly, the GIN index doesn't generally index single characters. It uses some rule to split the field into tokens. For example, for a text field, it might split the field into words (possibly with some normalization like case-folding and stemming) for an int array it might use the values in the array, etc. Jeremy asked specifically about btree_gin. The docs don't state that this index support ordered comparisons. This doesn't work if you split the field, so my guess is that it doesn't. For a single column it's basically a btree index with a more compact tid list. Dumping the contents of the index if hd seems to confirm this. For a btree_gin index spanning multiple columns I'm not sure. I would have expected each field to be a token, but it looks like both are stored together. So unless somebody more knowledgeable speaks up, I guess Jeremy will have to read the source. hp -- _ | Peter J. Holzer | we build much bigger, better disasters now |_|_) | | because we have much more sophisticated | | | hjp@hjp.at | management tools. __/ | http://www.hjp.at/ | -- Ross Anderson <https://www.edge.org/>
Вложения
On 2019-05-30 21:00:57 +0200, Peter J. Holzer wrote: > Firstly, the GIN index doesn't generally index single characters. It > uses some rule to split the field into tokens. For example, for a text > field, it might split the field into words (possibly with some > normalization like case-folding and stemming) for an int array it might > use the values in the array, etc. That was misleading: For a full text index you don't actually index the column. You index ts_vector(columne). It is ts_vector which does the tokenization, not the access method itself. hp -- _ | Peter J. Holzer | we build much bigger, better disasters now |_|_) | | because we have much more sophisticated | | | hjp@hjp.at | management tools. __/ | http://www.hjp.at/ | -- Ross Anderson <https://www.edge.org/>
Вложения
Will Hartung schrieb am 31.05.2019 um 00:11: > If you have 10M rows with a “STATUS” column of 1 or 2, and an index > on that column, then you have a 2 node index with a bazillion row > pointers. Some systems (I can’t speak to PG in this regard) > degenerate in this kind of use case since the index is more or less > designed to work great in unique identifiers than low cardinality > values. The representation of large record pointer lists may just not > be handled well as edge cases. What I very often do in theses cases is to create two partial indexes: One with "WHERE status = 1" and another with "WHERE STATUS = 2" including _another_ column in the index that I usually use together with the status column in the queries.
Jeremy's question is great, and really well presented. I can't answer his questions, but I am keenly interested in this subject as well. The links he provides lead to some really interesting and well-though-out pieces, well worth reading.I'm going to try restating things in my own way in hopes of getting some good feedback and a basic question:What are the best ways to index low cardinality values in Postgres?For an example, imagine an address table with 100M US street addresses with two character state abbreviations. So, say there are around 60 values in there (the USPS is the mail system for a variety of US territories, possessions and friends in the Pacific.) Okay, so what's the best index type for state abbreviation? For the sake of argument, assume a normal distribution so something like FM (Federated States of Micronesia) is on a tail end and CA or NY are a whole lot more common.A B-tree is obviously a pretty bad match for this sort of situation. It works, but B-trees are ideal for unique values, and super large for repeated values. Not getting into the details or Postgres specifics of various kinds of traditional B-trees. (I think B*?) Doesn't matter. You have a huge index because the index size is closely related to the number of rows, not the number of distinct values.Alternatively, you could set up partial indexes for the distinct values, like so:Running 10 Million PostgreSQL Indexes In Production (And Counting)Like Jeremy, I've wondered about GIN indexes for low-cardinality columns. Has anyone tried this out in PG 10 or 11? It sounds like a good idea. As I understand it, GIN indexes are something like a B-tree of unique values that link to another data structure, like a tree, bitmap, etc. So, in my imaginary example, there are 60 nodes for the state codes [internally there would be more for padding free nodes, evenly sized pages, etc....just pretend there are 60] and then linked to that, 60 data structures with the actual row references. Somehow.It can imagine things going quite well with a GIN or btree_gin. I can also imagine that the secondary data structure could get bloaty, slow, and horrible. I've worked with a system which uses bitmaps as the secondary structure (at least some of the time), and it can work quite well...not sure how it's implemented in Postgres.So, does anyone have any links or results about efficiently (space and/or time) indexing Boolean and other low-cardinality columns in Postgres? I'm on PG 11, but figure many are on 9.x or 10.x.References and results much appreciated.Thanks!
On 01/06/2019 14:52, Morris de Oryx wrote: [...] > For an example, imagine an address table with 100M US street addresses > with two character state abbreviations. So, say there are around 60 > values in there (the USPS is the mail system for a variety of US > territories, possessions and friends in the Pacific.) Okay, so what's > the best index type for state abbreviation? For the sake of argument, > assume a normal distribution so something like FM (Federated States of > Micronesia) is on a tail end and CA or NY are a whole lot more common. [...] I'd expect the distribution of values to be closer to a power law than the Normal distribution -- at very least a few states would have the most lookups. But this is my gut feel, not based on any scientific analysis! Cheers, Gavin
On 01/06/2019 14:52, Morris de Oryx wrote:
I'd expect the distribution of values to be closer to a power law than
the Normal distribution -- at very least a few states would have the
most lookups. But this is my gut feel, not based on any scientific
analysis!
On 2019-06-01 17:44:00 +1000, Morris de Oryx wrote: > Since I've been wondering about this subject, I figured I'd take a bit of time > and try to do some tests. I'm not new to databases or coding, but have been > using Postgres for less than two years. I haven't tried to generate large > blocks of test data directly in Postgres before, so I'm sure that there are > better ways to do what I've done here. No worries, this gave me a chance to > work through at least some of the questions/problems in setting up and running > tests. > > Anyway, I populated a table with 1M rows of data with very little in them, just > a two-character state abbreviation. There are only 59 values, and the > distribution is fairly even as I used random() without any tricks to shape the > distribution. So, each value is roughly 1/60th of the total row count. Not > realistic, but what I've got. > > For this table, I built four different kind of index and tried each one out > with a count(*) query on a single exact match. I also checked out the size of > each index. > > Headline results: > > Partial index: Smaller (as expeced), fast. > B-tree index: Big, fast. > GIN: Small, slow. > Hash: Large, slow. ("Large" may be exaggerated in comparison with a B-tree > because of my test data.) You didn't post any times (or the queries you timed), so I don't know what "fast" and "slow" mean. I used your setup to time select sum(num) from state_test where abbr = 'MA'; on my laptop (i5-7Y54, 16GB RAM, SSD, Pgsql 10.8) and here are the results: hjp=> select method, count(*), min(time_ms), avg(time_ms), percentile_cont(0.5) within group (order by time_ms) as median, max(time_ms) from state_test_times group by method order by 5; method | count | min | avg | median | max ---------+-------+--------+---------+--------+-------- Partial | 5 | 9.05 | 9.7724 | 9.185 | 12.151 B tree | 5 | 9.971 | 12.8036 | 10.226 | 21.6 GIN | 5 | 9.542 | 10.3644 | 10.536 | 10.815 Hash | 5 | 10.801 | 11.7448 | 11.047 | 14.875 All the times are pretty much the same. GIN is third by median, but the difference is tiny, and it is secondy by minium and average and even first by maximum. In this case all the queries do a bitmap scan, so the times are probably dominated by reading the heap, not the index. > method pg_table_size kb > Partial 401408 392 Kb > B tree 22487040 21960 Kb > GIN 1916928 1872 Kb > Hash 49250304 48096 Kb I get the same sizes. > Okay, so the partial index is smaller, basically proportional to the fraction > of the file it's indexing. So that makes sense, and is good to know. Yeah. But to cover all values you would need 59 partial indexes, which gets you back to the size of the full btree index. My test shows that it might be faster, though, which might make the hassle of having to maintain a large number of indexes worthwhile. > The hash index size is...harder to explain...very big. Maybe my tiny > strings? Not sure what size Postgres hashes to. A hash of a two > character string is likely about worst-case. I think that a hash index is generally a poor fit for low cardinality indexes: You get a lot of equal values, which are basically hash collisions. Unless the index is specifically designed to handle this (e.g. by storing the key only once and then a tuple list per key, like a GIN index does) it will balloon out trying to reduce the number of collisions. hp -- _ | Peter J. Holzer | we build much bigger, better disasters now |_|_) | | because we have much more sophisticated | | | hjp@hjp.at | management tools. __/ | http://www.hjp.at/ | -- Ross Anderson <https://www.edge.org/>
Вложения
On 2019-06-01 17:44:00 +1000, Morris de Oryx wrote:
> Since I've been wondering about this subject, I figured I'd take a bit of time
> and try to do some tests. I'm not new to databases or coding, but have been
> using Postgres for less than two years. I haven't tried to generate large
> blocks of test data directly in Postgres before, so I'm sure that there are
> better ways to do what I've done here. No worries, this gave me a chance to
> work through at least some of the questions/problems in setting up and running
> tests.
>
> Anyway, I populated a table with 1M rows of data with very little in them, just
> a two-character state abbreviation. There are only 59 values, and the
> distribution is fairly even as I used random() without any tricks to shape the
> distribution. So, each value is roughly 1/60th of the total row count. Not
> realistic, but what I've got.
>
> For this table, I built four different kind of index and tried each one out
> with a count(*) query on a single exact match. I also checked out the size of
> each index.
>
> Headline results:
>
> Partial index: Smaller (as expeced), fast.
> B-tree index: Big, fast.
> GIN: Small, slow.
> Hash: Large, slow. ("Large" may be exaggerated in comparison with a B-tree
> because of my test data.)
You didn't post any times (or the queries you timed), so I don't know
what "fast" and "slow" mean.
I used your setup to time
select sum(num) from state_test where abbr = 'MA';
on my laptop (i5-7Y54, 16GB RAM, SSD, Pgsql 10.8) and here are the
results:
hjp=> select method, count(*),
min(time_ms),
avg(time_ms),
percentile_cont(0.5) within group (order by time_ms) as median,
max(time_ms)
from state_test_times
group by method
order by 5;
method | count | min | avg | median | max
---------+-------+--------+---------+--------+--------
Partial | 5 | 9.05 | 9.7724 | 9.185 | 12.151
B tree | 5 | 9.971 | 12.8036 | 10.226 | 21.6
GIN | 5 | 9.542 | 10.3644 | 10.536 | 10.815
Hash | 5 | 10.801 | 11.7448 | 11.047 | 14.875
All the times are pretty much the same. GIN is third by median, but the
difference is tiny, and it is secondy by minium and average and even
first by maximum.
In this case all the queries do a bitmap scan, so the times are probably
dominated by reading the heap, not the index.
> method pg_table_size kb
> Partial 401408 392 Kb
> B tree 22487040 21960 Kb
> GIN 1916928 1872 Kb
> Hash 49250304 48096 Kb
I get the same sizes.
> Okay, so the partial index is smaller, basically proportional to the fraction
> of the file it's indexing. So that makes sense, and is good to know.
Yeah. But to cover all values you would need 59 partial indexes, which
gets you back to the size of the full btree index. My test shows that it
might be faster, though, which might make the hassle of having to
maintain a large number of indexes worthwhile.
> The hash index size is...harder to explain...very big. Maybe my tiny
> strings? Not sure what size Postgres hashes to. A hash of a two
> character string is likely about worst-case.
I think that a hash index is generally a poor fit for low cardinality
indexes: You get a lot of equal values, which are basically hash
collisions. Unless the index is specifically designed to handle this
(e.g. by storing the key only once and then a tuple list per key, like a
GIN index does) it will balloon out trying to reduce the number of
collisions.
hp
--
_ | Peter J. Holzer | we build much bigger, better disasters now
|_|_) | | because we have much more sophisticated
| | | hjp@hjp.at | management tools.
__/ | http://www.hjp.at/ | -- Ross Anderson <https://www.edge.org/>
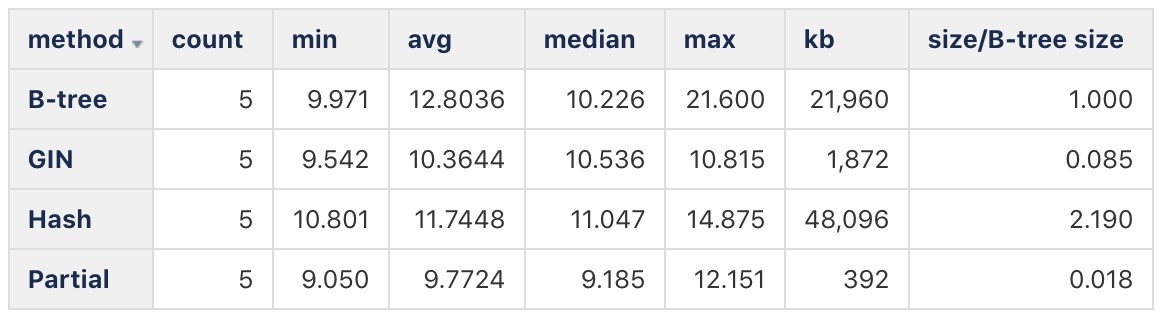
Peter, thanks a lot for picking up on what I started, improving it, and reporting back. I thought I was providing timing estimates from the EXPLAIN cost dumps. Seems not. Well, there's another thing that I've learned.Your explanation of why the hash index bloats out makes complete sense, I ought to have thought that.Can you tell me how you get timing results into state_test_times? I know how to turn on time display in psql, but I much prefer to use straight SQL. The reason for that is my production code is always run through a SQL session, not typing things into psql.On Sat, Jun 1, 2019 at 11:53 PM Peter J. Holzer <hjp-pgsql@hjp.at> wrote:On 2019-06-01 17:44:00 +1000, Morris de Oryx wrote:
> Since I've been wondering about this subject, I figured I'd take a bit of time
> and try to do some tests. I'm not new to databases or coding, but have been
> using Postgres for less than two years. I haven't tried to generate large
> blocks of test data directly in Postgres before, so I'm sure that there are
> better ways to do what I've done here. No worries, this gave me a chance to
> work through at least some of the questions/problems in setting up and running
> tests.
>
> Anyway, I populated a table with 1M rows of data with very little in them, just
> a two-character state abbreviation. There are only 59 values, and the
> distribution is fairly even as I used random() without any tricks to shape the
> distribution. So, each value is roughly 1/60th of the total row count. Not
> realistic, but what I've got.
>
> For this table, I built four different kind of index and tried each one out
> with a count(*) query on a single exact match. I also checked out the size of
> each index.
>
> Headline results:
>
> Partial index: Smaller (as expeced), fast.
> B-tree index: Big, fast.
> GIN: Small, slow.
> Hash: Large, slow. ("Large" may be exaggerated in comparison with a B-tree
> because of my test data.)
You didn't post any times (or the queries you timed), so I don't know
what "fast" and "slow" mean.
I used your setup to time
select sum(num) from state_test where abbr = 'MA';
on my laptop (i5-7Y54, 16GB RAM, SSD, Pgsql 10.8) and here are the
results:
hjp=> select method, count(*),
min(time_ms),
avg(time_ms),
percentile_cont(0.5) within group (order by time_ms) as median,
max(time_ms)
from state_test_times
group by method
order by 5;
method | count | min | avg | median | max
---------+-------+--------+---------+--------+--------
Partial | 5 | 9.05 | 9.7724 | 9.185 | 12.151
B tree | 5 | 9.971 | 12.8036 | 10.226 | 21.6
GIN | 5 | 9.542 | 10.3644 | 10.536 | 10.815
Hash | 5 | 10.801 | 11.7448 | 11.047 | 14.875
All the times are pretty much the same. GIN is third by median, but the
difference is tiny, and it is secondy by minium and average and even
first by maximum.
In this case all the queries do a bitmap scan, so the times are probably
dominated by reading the heap, not the index.
> method pg_table_size kb
> Partial 401408 392 Kb
> B tree 22487040 21960 Kb
> GIN 1916928 1872 Kb
> Hash 49250304 48096 Kb
I get the same sizes.
> Okay, so the partial index is smaller, basically proportional to the fraction
> of the file it's indexing. So that makes sense, and is good to know.
Yeah. But to cover all values you would need 59 partial indexes, which
gets you back to the size of the full btree index. My test shows that it
might be faster, though, which might make the hassle of having to
maintain a large number of indexes worthwhile.
> The hash index size is...harder to explain...very big. Maybe my tiny
> strings? Not sure what size Postgres hashes to. A hash of a two
> character string is likely about worst-case.
I think that a hash index is generally a poor fit for low cardinality
indexes: You get a lot of equal values, which are basically hash
collisions. Unless the index is specifically designed to handle this
(e.g. by storing the key only once and then a tuple list per key, like a
GIN index does) it will balloon out trying to reduce the number of
collisions.
hp
--
_ | Peter J. Holzer | we build much bigger, better disasters now
|_|_) | | because we have much more sophisticated
| | | hjp@hjp.at | management tools.
__/ | http://www.hjp.at/ | -- Ross Anderson <https://www.edge.org/>
Вложения
On 2019-06-02 09:10:25 +1000, Morris de Oryx wrote: > Peter, thanks a lot for picking up on what I started, improving it, and > reporting back. I thought I was providing timing estimates from the EXPLAIN > cost dumps. Seems not. Well, there's another thing that I've learned. The cost is how long the optimizer thinks it will take (in arbitrary units). But it's just an estimate, and estimates can be off - sometimes quite dramatically. To get the real timings with explain, use explain (analyze). I often combine this with buffers to get I/O stats as well: wdsah=> explain (analyze, buffers) select min(date) from facttable_stat_fta4 where partnerregion = 'USA' and sitcr4 = '7522'; ╔══════════════════════════════════════════════════════════════════════════════════════════════════════════════════════════════════════════════════════════════════════════════╗ ║ QUERY PLAN ║ ╟──────────────────────────────────────────────────────────────────────────────────────────────────────────────────────────────────────────────────────────────────────────────╢ ║ Aggregate (cost=694.23..694.24 rows=1 width=4) (actual time=7.568..7.568 rows=1 loops=1) ║ ║ Buffers: shared hit=3 read=148 dirtied=114 ║ ║ -> Index Scan using facttable_stat_fta4_sitcr4_partnerregion_idx on facttable_stat_fta4 (cost=0.57..693.09 rows=455width=4) (actual time=0.515..7.493 rows=624 loops=1) ║ ║ Index Cond: (((sitcr4)::text = '7522'::text) AND ((partnerregion)::text = 'USA'::text)) ║ ║ Buffers: shared hit=3 read=148 dirtied=114 ║ ║ Planning time: 0.744 ms ║ ║ Execution time: 7.613 ms ║ ╚══════════════════════════════════════════════════════════════════════════════════════════════════════════════════════════════════════════════════════════════════════════════╝ (7 rows) And when you don't need the costs, you can turn them off: wdsah=> explain (analyze, buffers, costs off) select min(date) from facttable_stat_fta4 where partnerregion = 'USA' and sitcr4= '7522'; ╔════════════════════════════════════════════════════════════════════════════════════════════════════════════════════════════════════════╗ ║ QUERY PLAN ║ ╟────────────────────────────────────────────────────────────────────────────────────────────────────────────────────────────────────────╢ ║ Aggregate (actual time=0.598..0.598 rows=1 loops=1) ║ ║ Buffers: shared hit=140 ║ ║ -> Index Scan using facttable_stat_fta4_sitcr4_partnerregion_idx on facttable_stat_fta4 (actual time=0.054..0.444 rows=624loops=1) ║ ║ Index Cond: (((sitcr4)::text = '7522'::text) AND ((partnerregion)::text = 'USA'::text)) ║ ║ Buffers: shared hit=140 ║ ║ Planning time: 0.749 ms ║ ║ Execution time: 0.647 ms ║ ╚════════════════════════════════════════════════════════════════════════════════════════════════════════════════════════════════════════╝ (7 rows) See https://www.postgresql.org/docs/current/sql-explain.html for details. > Can you tell me how you get timing results into state_test_times? In this case I just entered them manually (cut and paste from psql \timing output). If I wanted to repeat that test on another database, I would write a Python script (I'm sure you can do that in pgsql, too, but I feel more comfortable in Python). I don't think there is a way to get time timings in plain SQL. hp -- _ | Peter J. Holzer | we build much bigger, better disasters now |_|_) | | because we have much more sophisticated | | | hjp@hjp.at | management tools. __/ | http://www.hjp.at/ | -- Ross Anderson <https://www.edge.org/>
Вложения
I have been hoping for clearer direction from the community about specifically btree_gin indexes for low cardinality columns (as well as low cardinality multi-column indexes). In general there is very little discussion about this both online and in the docs. Rather, the emphasis for GIN indexes discussed is always on full text search of JSON indexing, not btree_gin indexes.However, I have never been happy with the options open to me for indexing low cardinality columns and was hoping this could be a big win. Often I use partial indexes as a solution, but I really want to know how many use cases btree_gin could solve better than either a normal btree or a partial index.
Here are my main questions:1."The docs say regarding *index only scans*: The index type must support index-only scans. B-tree indexes always do. GiST and SP-GiST indexes support index-only scans for some operator classes but not others. Other index types have no support. The underlying requirement is that the index must physically store, or else be able to reconstruct, the original data value for each index entry. As a counterexample, GIN indexes cannot support index-only scans because each index entry typically holds only part of the original data value."This is confusing to say "B-tree indexes always do" and "GIN indexes cannot support index-only scans", when we have a btree_gin index type. Explanation please ???
Is it true that for a btree_gin index on a regular column, "each index entry typically holds only part of the original data value"? Do these still not support index only scans? Could they? I can't see why they shouldn't be able to for a single indexed non-expression field?
2.Lack of index only scans is definitely a downside. However, I see basically identical performance, but way less memory and space usage, for gin indexes. In terms of read-only performance, if index only scans are not a factor, why not always recommend btree_gin indexes instead of regular btree for low cardinality fields, which will yield similar performance but use far, far less space and resources?
3.This relates to 2. I understand the write overhead can be much greater for GIN indexes, which is why the fastupdate feature exists. But again, in those discussions in the docs, it appears to me they are emphasizing that penalty more for full text or json GIN indexes. Does the same overhead apply to a btree_gin index on a regular column with no expressions?
Jeff Janes <jeff.janes@gmail.com> writes: > On Fri, May 24, 2019 at 11:26 AM Jeremy Finzel <finzelj@gmail.com> wrote: >> I have been hoping for clearer direction from the community about >> specifically btree_gin indexes for low cardinality columns (as well as low >> cardinality multi-column indexes). In general there is very little >> discussion about this both online and in the docs. Rather, the emphasis >> for GIN indexes discussed is always on full text search of JSON indexing, >> not btree_gin indexes. I just wanted to mention that Jeremy and I had a bit of hallway-track discussion about this at PGCon. The core thing to note is that the GIN index type was designed to deal with data types that are subdividable and you want to search for individual component values (array elements, lexemes in a text document, etc). The btree_gin extension abuses this by just storing the whole values as if they were components. AFAIR, the original idea for writing both btree_gin and btree_gist was to allow creating a single multicolumn index that covers both subdividable and non-subdividable columns. The idea that btree_gin might be used on its own wasn't really on the radar, I don't think. However, now that GIN can compress multiple index entries for the same component value (which has only been true since 9.4, whereas btree_gin is very much older than that) it seems like it does make sense to use btree_gin on its own for low-cardinality non-subdividable columns. And that means that we ought to consider non-subdividable columns as fully legitimate, not just a weird corner usage. So in particular I wonder whether it would be worth adding the scaffolding necessary to support index-only scan when the GIN opclass is one that doesn't subdivide the data values. That leaves me quibbling with some points in Jeff's otherwise excellent reply: > For single column using a btree_gin operator, each index entry holds the > entire data value. But the system doesn't know about that in any useful > way, so it doesn't implement index only scans, other than in the special > case where the value does not matter, like a 'count(*)'. Conceptually > perhaps this could be fixed, but I don't see it happening. Since an > index-only scan is usually not much good with only a single-column index, I > don't see much excitement to improve things here. I'm confused by this; surely IOS is useful even with a single-column index? Avoiding trips to the heap is always helpful. > If if there is more than > one column in the index, then for GIN the entire value from both columns > would not be stored in the same index entry, so in this case it can't use > an index-only scan even conceptually to efficiently fetch the value of one > column based on the supplied value of another one. Yeah, that is a nasty issue. You could do it, I think, by treating the additional column as being queried even though there is no WHERE constraint on it --- but that would lead to scanning all the index entries which would be very slow. Maybe still faster than a seqscan though? But I suspect we'd end up wanting to extend the notion of "IOS" to say that GIN can only return columns that have an indexable constraint, which is a little weird. At the very least we'd have to teach gincostestimate about this, or we could make very bad plan choices. Anyway, I said to Jeremy in the hallway that it might not be that hard to bolt IOS support onto GIN for cases where the opclass is a non-subdividing one, but after looking at the code I'm less sure about that. GIN hasn't even got an "amgettuple" code path, just "amgetbitmap", and a big part of the reason why is the need to merge results from the fastupdate pending list with results from the main index area. Not sure how we could deal with that. > GIN indexes over btree_gin operators do not support inequality or BETWEEN > queries efficiently. Are you sure about that? It's set up to use the "partial match" logic, which is certainly pretty weird, but it does have the potential for handling inequalities efficiently. [ pokes at it ... ] Hm, looks like it does handle individual inequality conditions reasonably well, but it's not smart about the combination of a greater-than and a less-than constraint on the same column. It ends up scanning all the entries satisfying the one condition, plus all the entries satisfying the other, then intersecting those sets --- which of course comprise the whole table :-(. I think maybe this could be made better without a huge amount of work though. > ... I do recall that > the replay of GIN WAL onto a standby was annoying slow, but I haven't > tested it with your particular set up and not in the most recent versions. A quick look at the commit log says that some work was done in this area for 9.4, and more in v12, but I've not tried to measure the results. Anyway, the larger point here is that right now btree_gin is just a quick hack, and it seems like it might be worth putting some more effort into it, because the addition of duplicate-compression changes the calculus for whether it's useful. regards, tom lane
>As an example, we're dealing with millions of rows where we often want to find or summarize by a category value. So, maybe 6-10 categories that are used in various queries. It's not realistic for us to anticipate every field combination the category field is going to be involved in to lay down multi-column indexes everywhere.
Apologies if I’ve missed this somewhere else in the thread, but I’ve not seen anyone suggest that bloom indexes[1] be thrown into the mix.
Depending on your use case, you might be able to replace many multi-column btree indexes with a single bloom index, optimizing its size vs. performance using the “length” parameter. You could even reduce the number of bits generated for low cardinality columns to 1, which should reduce the number of false positives that are later removed by a condition recheck.
Maybe there’s a good reason for their omission, but I’d like to learn this I’m completely off the mark!
Steve.
> In the case of a single column with a small set of distinct values over a large set of rows, how would a Bloom filter bepreferable to, say, a GIN index on an integer value? I don't think it would - it's probably better suited to the multi-column case you described previously. > I have to say, this is actually a good reminder in my case. We've got a lot of small-distinct-values-big-rows columns.For example, "server_id", "company_id", "facility_id", and so on. Only a handful of parent keys with many millionsof related rows. Perhaps it would be conceivable to use a Bloom index to do quick lookups on combinations of suchvalues within the same table. I haven't tried Bloom indexes in Postgres, this might be worth some experimenting. Yes, this is more like the use case I was thinking of. Steve.
On Sun, Jun 2, 2019 at 4:07 PM Tom Lane <tgl@sss.pgh.pa.us> wrote: > Anyway, I said to Jeremy in the hallway that it might not be that > hard to bolt IOS support onto GIN for cases where the opclass is > a non-subdividing one, but after looking at the code I'm less sure > about that. GIN hasn't even got an "amgettuple" code path, just > "amgetbitmap", and a big part of the reason why is the need to merge > results from the fastupdate pending list with results from the main > index area. Not sure how we could deal with that. I suspect that GIN also avoids several other problems by only offer an "amgetbitmap", and not an "amgettuple". For example, it doesn't have to worry about things like numeric display scale, where a datum is substantively different to another datum, while still being equal according to opclass semantics (this is an example that I end up citing in many discussions about indexing). I bet that there are a few more of those beyond those two, that I haven't thought about. > Anyway, the larger point here is that right now btree_gin is just a quick > hack, and it seems like it might be worth putting some more effort into > it, because the addition of duplicate-compression changes the calculus > for whether it's useful. There was also discussion about making nbtree support deduplication during the hallway track. Jim Finnerty reminded me that there is a patch from Anastasia that did deduplication in nbtree that didn't go anywhere. Heikki independently talked about the possibility that he would work on this project in the next release, without being prompted by me. I think that the fact that nbtree sorts duplicate entries in heap TID order these days makes that worth looking into again. We can use something like GIN's varbyte encoding process to compress duplicates effectively. A lot of the problems (the numeric display scale problem, pg_upgrade) can be avoided by defining deduplication as something that happens on a best-effort basis. This is not the case within GIN, where it's impossible for the main entry tree to have duplicates without at least storing them in a posting list. -- Peter Geoghegan
Jeff Janes <jeff.janes@gmail.com> writes:
> On Fri, May 24, 2019 at 11:26 AM Jeremy Finzel <finzelj@gmail.com> wrote:
>> I have been hoping for clearer direction from the community about
>> specifically btree_gin indexes for low cardinality columns (as well as low
>> cardinality multi-column indexes). In general there is very little
>> discussion about this both online and in the docs. Rather, the emphasis
>> for GIN indexes discussed is always on full text search of JSON indexing,
>> not btree_gin indexes.
I just wanted to mention that Jeremy and I had a bit of hallway-track
discussion about this at PGCon. The core thing to note is that the GIN
index type was designed to deal with data types that are subdividable
and you want to search for individual component values (array elements,
lexemes in a text document, etc). The btree_gin extension abuses this
by just storing the whole values as if they were components. AFAIR,
the original idea for writing both btree_gin and btree_gist was to allow
creating a single multicolumn index that covers both subdividable and
non-subdividable columns. The idea that btree_gin might be used on its
own wasn't really on the radar, I don't think.
However, now that GIN can compress multiple index entries for the same
component value (which has only been true since 9.4, whereas btree_gin
is very much older than that) it seems like it does make sense to use
btree_gin on its own for low-cardinality non-subdividable columns.
And that means that we ought to consider non-subdividable columns as
fully legitimate, not just a weird corner usage. So in particular
I wonder whether it would be worth adding the scaffolding necessary
to support index-only scan when the GIN opclass is one that doesn't
subdivide the data values.
That leaves me quibbling with some points in Jeff's otherwise excellent
reply:
> For single column using a btree_gin operator, each index entry holds the
> entire data value. But the system doesn't know about that in any useful
> way, so it doesn't implement index only scans, other than in the special
> case where the value does not matter, like a 'count(*)'. Conceptually
> perhaps this could be fixed, but I don't see it happening. Since an
> index-only scan is usually not much good with only a single-column index, I
> don't see much excitement to improve things here.
I'm confused by this; surely IOS is useful even with a single-column
index? Avoiding trips to the heap is always helpful.
> GIN indexes over btree_gin operators do not support inequality or BETWEEN
> queries efficiently.
Are you sure about that? It's set up to use the "partial match" logic,
which is certainly pretty weird, but it does have the potential for
handling inequalities efficiently. [ pokes at it ... ] Hm, looks like
it does handle individual inequality conditions reasonably well, but
it's not smart about the combination of a greater-than and a less-than
constraint on the same column. It ends up scanning all the entries
satisfying the one condition, plus all the entries satisfying the other,
then intersecting those sets --- which of course comprise the whole table
:-(. I think maybe this could be made better without a huge amount of
work though.
Anyway, the larger point here is that right now btree_gin is just a quick
hack, and it seems like it might be worth putting some more effort into
it, because the addition of duplicate-compression changes the calculus
for whether it's useful.
- It's selective enough that having *some kind of index* actually will significantly speed up queries vs. a sequential scan
- There are too many values to use partial indexes easily without it becoming a kind of maintenance nightmare
- Table has 127 million rows, including a toast field. The table is 270GB
- The filter is on a field with only 16 unique values.
- The actual filter condition is filtering a join to 4 of the 16 unique values
- Memory for join using btree: Buffers: shared hit=12 read=328991
- Memory for join using gin: Buffers: shared hit=12 read=13961